Covid-19: Spotting the Negative Patterns
In the second half of Covid, we were asked by a local authority to help them suppress Covid outbreaks.
In the absence of mandates and with dwindling funds, they were reliant on their mobile emergency response teams. The teams would head into areas where outbreaks were occurring, inform the people who lived there of their increasing risk, and then offer strategies designed to reduce infection and transmission.
It was like trying to put out a volcano with a bucket of water. No matter where their teams went, they felt they were not having the impact needed.
The Missing Data
When we asked them how they were deciding on where to go, they showed us a heatmap. Quite rationally, they were geo-mapping PCR and LFD test data and then heading to the areas which lit up on the map. Their programme of onsite testing illuminated the map even more. So, often they were in these areas for several days at a time.
We linked their data to five other datasets and what we discovered was that the people being targeted by the emergency response teams were in fact the most affluent and educated segments of the local population.
We knew that this had to be inaccurate.
Our hypothesis was that there was dark matter in the data. In other words, people who should be showing up weren’t.
We suggested that, instead of mapping Covid levels in the population, they were mapping locations where people were most likely to report the outcome of a test they had taken. The data was biased.
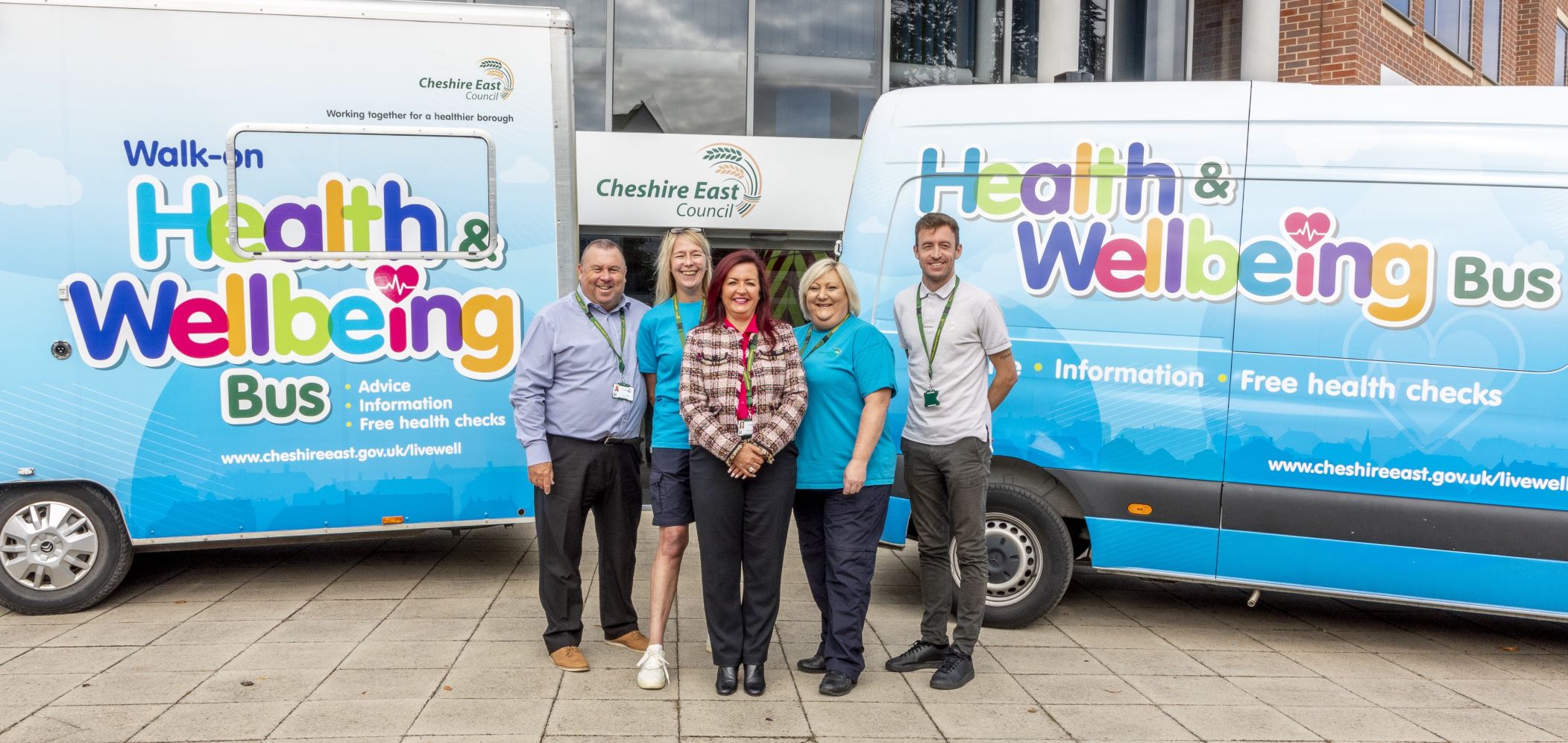
How We Solved This
We brokered a deal with the UK Health Security Agency (UKHSA) and replaced the biased reported test data with new data – taken from routine sampling of Covid viral load in waste water. This was highly accurate, and we were able to pinpoint case levels down to small geographic areas.
We built a Covid dashboard which lit up a very different map. Unsurprisingly, the areas which lit up now were not the same areas they’d been going out to.
Not only this, but we combined risk (Covid concentration) with vulnerability (level of immunity and predicted behavioural response to evasive measures) ranked by SOA and ward. The picture changed in real time, allowing a more accurate picture of where they needed to be.
The team were now able to leverage the greatest impact from the resource they had.